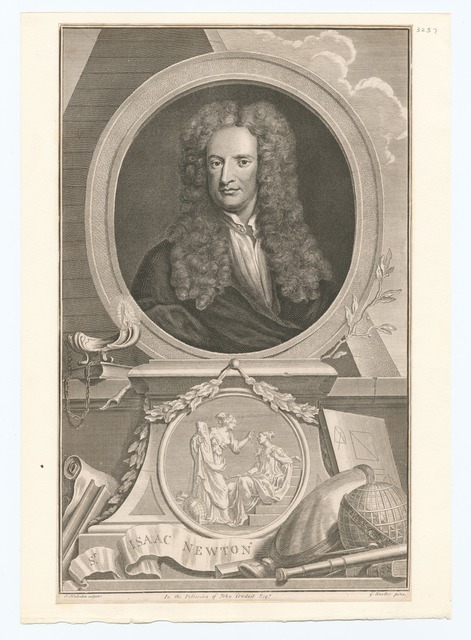
Since the mid-19th century, science has been a discipline that requires a credential from a college (for a BA) and from a university (for an MA or PhD). But it was not always so. From the Enlightenment era (late 1600s) onwards, talented, engaged, amateur gentlemen could be what was called “natural philosopher”, or in modern terms, “citizen scientist”. That meant they studied things in the natural world, wrote papers and joined clubs and societies. These men were almost always rich because their research and experiments were self-funding. And no credential required. As long as one had the money, the time and the inclination, he could join this elite cadre of thinkers. (Oh and a penis. Sorry for my crudeness, but “natural philosophers” were almost exclusively men, women’s brains not yet being widely acknowledged.)
In the mid 19th century and into the 20th century, credentialing became a requirement for becoming a scientist. This development was driven, at least in part, by the expense of lab equipment, infrastructure, and materials. As science became more complex, so did the equipment necessary to study it. No longer could a wealthy gentleman set up a working lab at home. As complexity grew, so did the skills that you had to master. This then created an accepted body of knowledge you had to master to be capable of studying science.
Now, early in the 21st century, we are seeing a re-branding of the “citizen scientist” as a “citizen data scientist”.
Here’s what the old and new “citizen scientist” don’t share (mercifully):
- The de facto requirement to be a man
- A requirement to be wealthy and leisured
Here’s what they do have in common:
- A requirement to be passionate about a particular subject (in this case, all things data).
- A willingness (and desire) to share knowledge with other like minded people.
- No particular certification or accreditation to be credible.
- Accessibility of the machinery and materials to study and experiment.
- A wide open field, where anyone with time, energy, discipline and passion can make his/her mark.
The wide open nature of the data world has made me think a lot about the descriptor “citizen data scientist”. Appending the word “scientist” invokes the specter of diplomas, and triggers “impostor syndrome” for many people, even if they do hold a credential (but not the credential of “data scientist”). Calling everyone a “scientist” also minimizes the distinction between the skills of a trained data scientist and those of a talented, engaged amateur. I do not consider myself a “citizen data scientist“. I don’t have the mathematical skills required to be a credentialed data scientist. I don’t want to misrepresent myself by using this title.
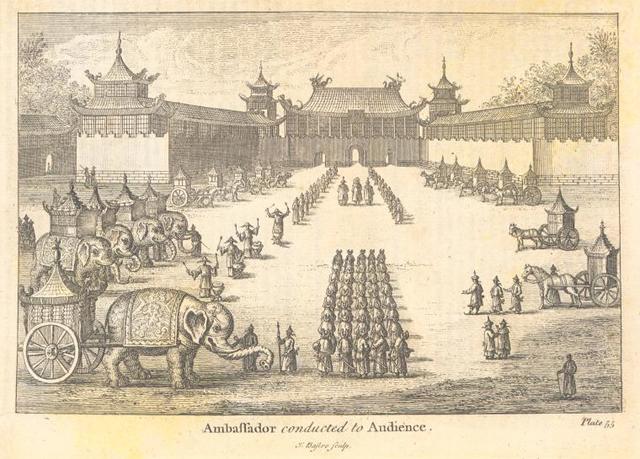
I do consider myself a “citizen data ambassador”. I work to bring people into the new world of Microsoft’s Power Platform (Power BI, Flow, and Power Apps) by showing that even a person who cried through every math class she ever took (that’s me!) can develop the skills to use data – based business intelligence tools.
There are lots of “data ambassadors” out there. We are smart, passionate (and fun) professionals working to bring other professionals into the world of data in general and Microsoft’s Power Platform specifically. Our skills are not as specifically and narrowly defined as those of a credentialed data scientist. We specialize in different areas: visualizing data, analyzing data, automating data, sharing data, and many more. We are unconstrained by any boundaries other than our own energy, time, and enthusiasm. We make a living using data in a variety of ways. We may only focus on one particular aspect or one particular audience. The one thing we have in common is we love helping other people learn to work with data.
So please don’t call me a “citizen data scientist”. I have mad respect for data scientists and their knowledge. But I have not (and probably could not) earn that degree. But that fact doesn’t diminish what I do know and what I can offer. I can show people how to get started using Microsoft’s Power Platform. I can share the resources that I use to increase my own skills. I can encourage people to reject perceived blockers to mastery: “I am too old”, “I am technically inept”. I can serve as a role model for someone who needs one. I am a “Data Ambassador”.